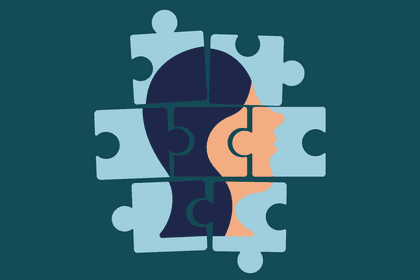
Landscaping international longitudinal datasets
This report identifies longitudinal datasets from around the world and highlights some that offer promising opportunities for mental health research.
As part of Wellcome’s vision for a world in which no one is held back by mental health problems, we commissioned an international landscaping survey in 2022 of existing longitudinal datasets that can advance science on how the brain, body and environment interact to influence the course of depression, anxiety and psychosis.
What are longitudinal datasets?
A longitudinal dataset contains information that has been collected repeatedly over time about a group of people. Longitudinal datasets can enable research on the patterns of change across long periods of time.
Introduction to the report
Following an extensive search for longitudinal datasets across the globe and various sectors, we present a comprehensive review of the existing richness of mental health data and analyse a selection of large ongoing datasets to uncover innovative resources that can support future mental health research.
Main objectives
1. Review the richness
The first objective of this research was to review the richness of all identified longitudinal datasets with mental health data that can be used for finding new and improved ways to predict, identify and intervene as early as possible for mental conditions such as depression, anxiety and psychosis.
2. Establish areas of enrichment
The second objective was to establish areas of enrichment using a selection of ongoing longitudinal datasets. Enrichment would be through new data collection or recruitment of participants. These datasets were selected based on:
- large sample sizes (8,000 participants or more at inception)
- granular assessments
- mental health data collected (or could be collected) from participants aged between 14 and 30 years.
Ultimately, this research aimed to make longitudinal datasets more discoverable, encourage their use by mental health researchers and other data users, highlight their richness and consider opportunities for further enrichment.
Methodology
We created a partnership of academic institutions (King’s College London), charities (MQ Mental Health Research), non-profit organisations (the Open Data Institute) and Lived Experience Expert (LEE) groups to complete this project. We also worked with a range of national and international collaborators.
We divided the work for this project into six stages:
- Stage 1: searching for datasets and identifying longitudinal datasets
- Stage 2: scoping for richness of datasets
- Stage 3: screening for selection criteria (for example, sample size, age of participants, ongoing status of mental health data)
- Stage 4: reviewing selected datasets for enrichment (for example, attrition, representativeness, data access, mental healh measures)
- Stage 5: assessing areas of enrichment
- Stage 6: reporting.
Input from various stakeholders was gathered as part of a Theory of Change (ToC) process throughout the project. View the full report about the ToC process from MQ.
Key findings
Through our surveying, we identified:
- over 3,000 longitudinal datasets worldwide - 25% from the Americas, 25% from Africa, the Middle East and Asia, 46% from Europe and the Pacific, and 4% cross-regions. These datasets came from 146 different countries. Countries without longitudinal datasets were mostly small Low- and Middle-Income Countries (LMICs)
- richness in several longitudinal datasets that fell under four categories: richness in mental health measures, value in targeted populations, diversity of data and mental health embedded in a wider context
- each geographical location provided specific value, such as routinely collected data in Europe, long-standing cohorts in the Americas and datasets embedded in social context in Africa
- a lack of ongoing studies with large sample sizes, granular assessments and mental health data when participants are between 14 and 30 years: only 100 of the longitudinal datasets we identified (3%) met these criteria for further consideration and of those, only one in 10 primarily focused on mental health
- enrichment of these longitudinal datasets include preservation and expansion of targeted populations, improvement of measurement and collection of new data, infrastructure and connectivity across datasets and promotion of LEE involvement, community engagement and input from service users.
Pockets of value
During our worldwide review of longitudinal datasets, we noted the richness of several datasets and the opportunities they offer for transformative mental health research. We grouped the richness into 19 'pockets of value', and then grouped these pockets of value into four wider categories.
- Strong measurement of depression and anxiety
- Valuable resources for psychosis research
- Other mental health conditions
- Mental health across the life course
- High-risk groups
- Under-represented groups
- Populations from under-represented locations
- Ageing populations
- Value in the long run
- Factors contributing to mental health conditions
- Neuroimaging data
- Wearables and phone apps
- Biological and genetic data
- Routinely collected data
- Mental health within social context
- The impact of the Covid-19 pandemic
- Connectivity between datasets
- Natural disasters and geopolitical factors
- Interventions embedded in longitudinal datasets
Challenges
We cannot guarantee that we turned over every stone and we are still finding datasets past the end of the project. Yet, we remain confident we unearthed all relevant datasets for mental health research given our thorough search, our extensive networks of collaborators in the field and our prominent presence on social media where we disseminated the project beyond the UK and mental health research communities.
Our search for longitudinal datasets could have further explored fields outside mental health and in sectors beyond academia. While we identified several datasets that focused on physical health and economic factors, our outreach within the community of researchers in disciplines outside mental health was limited.
Mapping the world for longitudinal datasets, and reviewing their value, is not an exact science. The estimates reported do not provide ‘true’ figures but were instead meant to produce an appreciation of global resources for mental health research. Interpretation of this report should focus on relative rather than absolute values.
Recommendations
While we found several especially rich datasets for mental health research, we did not identify one perfect source of data. We noticed, however, groups of datasets that could complement each other and increase their value by joining forces. Rather than overburdening participants of a single study by collecting too much data too frequently, researchers and funders could consider concerted efforts in optimising the value of groups of especially promising studies.
We recommend a coordinated approach to funding longitudinal research and a resolute will to improve the discoverability of existing datasets in order to maximise the financial, time and resource investment made thus far in establishing and maintaining longitudinal datasets.
Contact
For inquiries on:
- the overall report, contact Louise Arseneault (King’s College London)
- the Theory of Change workshop and Lived Experience Experts involvement, contact Lea Milligan (MQ)
- global coverage of the report, contact Crick Lund (Centre for Global Mental Health).